Statistics and Data Science Seminars
Apr 24, 2024 02:00 PM
ZOOM
Speaker: Dr. Shuoyang Wang (Assistant Professor, University of Louisville)
Title: Inference on High-dimensional Mediation Analysis with Convoluted Confounding via Deep Neural Networks
Abstract: Traditional linear mediation analysis has inherent limitations when it comes to handling high-dimensional mediators. Particularly, accurately estimating and rigorously inferring mediation effects is challenging, primarily due to the intertwined nature of the mediator selection issue. Despite recent developments, the existing methods are inadequate for addressing the complex relationships introduced by confounders. To tackle these challenges, we propose a novel approach called DP2LM (Deep neural network based Penalized Partially Linear Mediation). DP2LM incorporates deep neural network techniques to account for nonlinear effects in confounders and utilizes the penalized partially linear model to accommodate high dimensionality. In addition, to address the influence of outliers on mediation effects, we present an enhanced version of DP2LM called QDP2LM (Quantile Deep Neural Network-based Penalized Partially Linear Mediation). QDP2LM builds upon DP2LM and provides a comprehensive assessment of mediation effects across various quantiles. Unlike most existing works that concentrate on mediator selection, our methods prioritize estimation and inference on mediation effects. Specifically, we develop test procedures for testing the direct and indirect mediation effects. Theoretical analysis shows that the proposed procedures control type I error rates for hypothesis testing on mediation effects. Numerical studies show that the proposed methods outperform existing approaches under a variety of settings, demonstrating their versatility and reliability as modeling tools for complex data. Our application of the proposed methods to study DNA methylation's mediation effects of childhood trauma on cortisol stress reactivity reveals previously undiscovered relationships through a comprehensive analysis.
DMS Statistics and Data Science Seminar
Apr 17, 2024 02:00 PM
354 Parker Hall
Speaker: Dr. Shujie Ma (University of California at Riverside)
Title: Causal Inference on Quantile Dose-response Functions via Local ReLU Least Squares Weighting
Abstract: In this talk, I will introduce a novel local ReLU network least squares weighting method to estimate quantile dose-response functions in observational studies. Unlike the conventional inverse propensity weighting (IPW) method, we estimate the weighting function involved in the treatment effect estimator directly through local ReLU least squares optimization. The proposed method takes advantage of ReLU networks applied for the baseline covariates with increasing dimension to alleviate the dimensionality problem while retaining flexibility and local kernel smoothing for the continuous treatment to precisely estimate the quantile dose-response function and prepare for statistical inference. Our method enjoys computational convenience, scalability, and flexibility. It also improves robustness and numerical stability compared to the conventional IPW method. We show that the ReLU networks can break the notorious `curse of dimensionality' when the weighting function belongs to a newly introduced smoothness class. We also establish the convergence rate for the ReLU network estimator and the asymptotic normality of the proposed estimator for the quantile dose-response function. We further propose a multiplier bootstrap method to construct confidence bands for quantile dose-response functions. The finite sample performance of our proposed method is illustrated through simulations and a real data application.
DMS Statistics and Data Science Seminar
Apr 10, 2024 02:00 PM
354 Parker Hall
Speaker: Dr. Ted Westling (Assistant Professor, University of Massachusetts Amherst)
Title: Consistency of the bootstrap for asymptotically linear estimators based on machine learning
Abstract: The bootstrap is a popular method of constructing confidence intervals due to its ease of use and broad applicability. Theoretical properties of bootstrap procedures have been established in a variety of settings. However, there is limited theoretical research on the use of the bootstrap in the context of estimation of a differentiable functional in a nonparametric or semiparametric model when nuisance functions are estimated using machine learning. In this article, we provide general conditions for consistency of the bootstrap in such scenarios. Our results cover a range of estimator constructions, nuisance estimation methods, bootstrap sampling distributions, and bootstrap confidence interval types. We provide refined results for the empirical bootstrap and smoothed bootstraps, and for one-step estimators, plug-in estimators, empirical mean plug-in estimators, and estimating equations-based estimators. We illustrate the use of our general results by demonstrating the asymptotic validity of bootstrap confidence intervals for the average density value and G-computed conditional mean parameters, and compare their performance in finite samples using numerical studies. Throughout, we emphasize whether and how the bootstrap can produce asymptotically valid confidence intervals when standard methods fail to do so.
This is joint work with UMass Amherst Statistics PhD student Zhou Tang. A preprint of the paper is available here: https://arxiv.org/abs/2404.03064.
DMS Statistics and Data Science Seminar
Apr 03, 2024 02:00 PM
ZOOM
Speaker: Dr. Panpan Zhang (Assistant Professor, Vanderbilt University Medical Center)
Title: Challenges and Opportunities for Longitudinal Analysis of Neurodegenerative Disorders
Abstract: Alzheimer's disease (AD) and Parkinson's disease (PD) are chronic neurodegenerative disorders that gradually destroy memory, thinking skills, and mobility, causing significant impacts on life quality and economic burden. Longitudinal analysis is a promising tool that helps clinicians and neuroscientists better understand changes in the characteristics of the target population over the continuum of AD (or PD) progression. However, the lengthy course of development of such diseases poses many challenges in biostatistical studies. In this presentation, I will introduce two recent projects respectively focusing on missing covariate problems and mismatching time scale problems arising from the longitudinal modeling of AD and PD. I will showcase the novelty of the proposed methods, but also discuss their limitations and potential improvements. The applications in these two projects are primarily based on the open data from the Parkinson's Progression Markers Initiative (PPMI) and the Alzheimer's Disease Neuroimaging Initiative (ADNI).
DMS Statistics and Data Science Seminar
Mar 20, 2024 02:00 PM
354 Parker Hall
Speaker: Dr. Linbo Wang (University of Toronto)
Title: Sparse Causal Learning
Abstract: In many observational studies, researchers are interested in studying the effects of multiple exposures on the same outcome. Unmeasured confounding is a key challenge in these studies as it may bias the causal effect estimate. To mitigate the confounding bias, we introduce a novel device, called the synthetic instrument, to leverage the information contained in multiple exposures for causal effect identification and estimation. We show that under linear structural equation models, the problem of causal effect estimation can be formulated as an \(\ell_0\)-penalization problem, and hence can be solved efficiently using off-the-shelf software. Simulations show that our approach outperforms state-of-art methods in both low-dimensional and high-dimensional settings. We further illustrate our method using a mouse obesity dataset.
Bio: Linbo Wang is an assistant professor in the Department of Statistical Sciences and the Department of Computer and Mathematical Sciences, University of Toronto. He is also a faculty affiliate at the Vector Institute, a CANSSI Ontario STAGE program mentor, and an Affiliate Assistant Professor in the Department of Statistics, University of Washington, and Department of Computer Science, University of Toronto. Prior to these roles, he was a postdoc at Harvard T.H. Chan School of Public Health. He obtained his Ph.D. from the University of Washington. His research interest is centered around causality and its interaction with statistics and machine learning.
DMS Statistics and Data Science Seminar
Mar 13, 2024 02:00 PM
354 Parker Hall
Speaker: Dr. Sathyanarayanan Aakur, Assistant Professor from Auburn CSSE.
Title: Towards Multimodal Open World Event Understanding with Neuro Symbolic Reasoning.
Abstract: Deep learning models for multimodal understanding have taken great strides in tasks such as event recognition, segmentation, and localization. However, there appears to be an implicit closed world assumption in these approaches; i.e., they assume that all observed data is composed of a static, known set of objects (nouns), actions (verbs), and activities (noun+verb combination) that are in 1:1 correspondence with the vocabulary from the training data. One must account for every eventuality when training these systems to ensure their performance in real-world environments. In this talk, I will present our recent efforts to build open-world understanding models that leverage the general-purpose knowledge embedded in large-scale knowledge bases for providing supervision using a neuro-symbolic framework based on Grenander’s Pattern Theory formalism. Then I will talk about how this framework can be extended to abductive reasoning for natural language inference and commonsense reasoning for visual understanding. Finally, I will briefly present some results from the bottom-up neural side of open-world event perception that helps navigate clutter and provides cues for the abductive reasoning frameworks.
DMS Statistics and Data Science Seminar
Nov 15, 2023 02:00 PM
354 Parker Hall
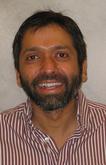
DMS Statistics and Data Science Seminar
Nov 08, 2023 02:00 PM
ZOOM
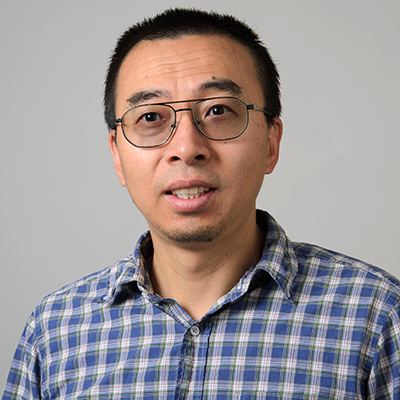
Speaker: Dr. HaiYing Wang (University of Connecticut)
Title: Rare Events Data and Maximum Sampled Conditional Likelihood
Abstract: We show that the available information about unknown parameters in rare events data is only tied to the relatively small number of cases, which justifies the usage of negative sampling. However, if the negative instances are subsampled to the same level of the positive cases, there is information loss. We derive an optimal sampling probability for the inverse probability weighted (IPW) estimator to minimize the information loss. We further we propose a likelihood-based estimator to further improve the estimation efficiency, and show that the improved estimator has the smallest asymptotic variance among a large class of estimators. It is also more robust to pilot misspecification. The likelihood-based estimator is also generalized to a class of models beyond binary response models. We validate our approach on simulated data, the MNIST data, and a real click-through rate dataset with more than 0.3 trillion instances.
DMS Statistics and Data Science Seminar
Nov 01, 2023 02:00 PM
354 Parker Hall
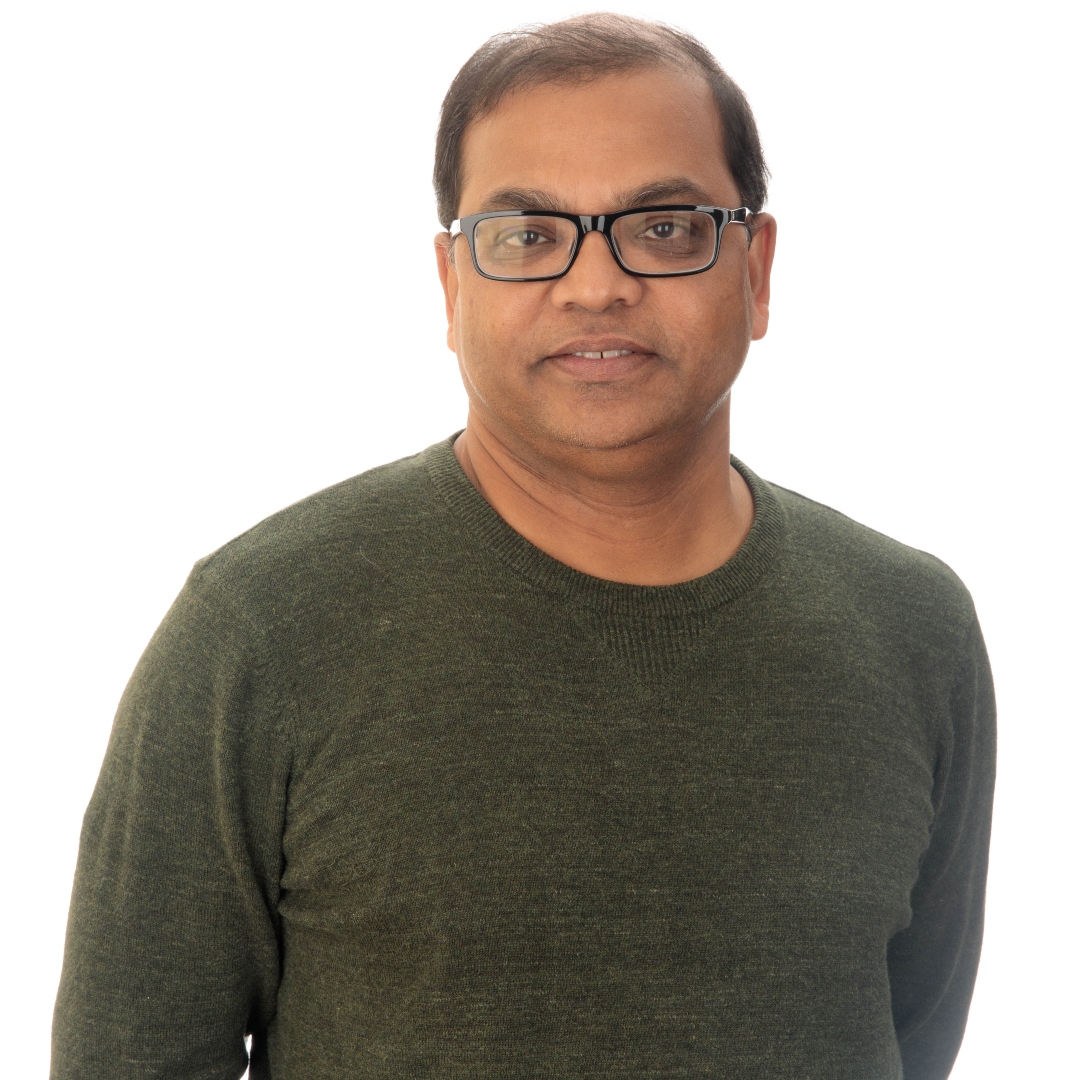
DMS Statistics and Data Science Seminar
Oct 25, 2023 02:00 PM
354 Parker Hall / ZOOM
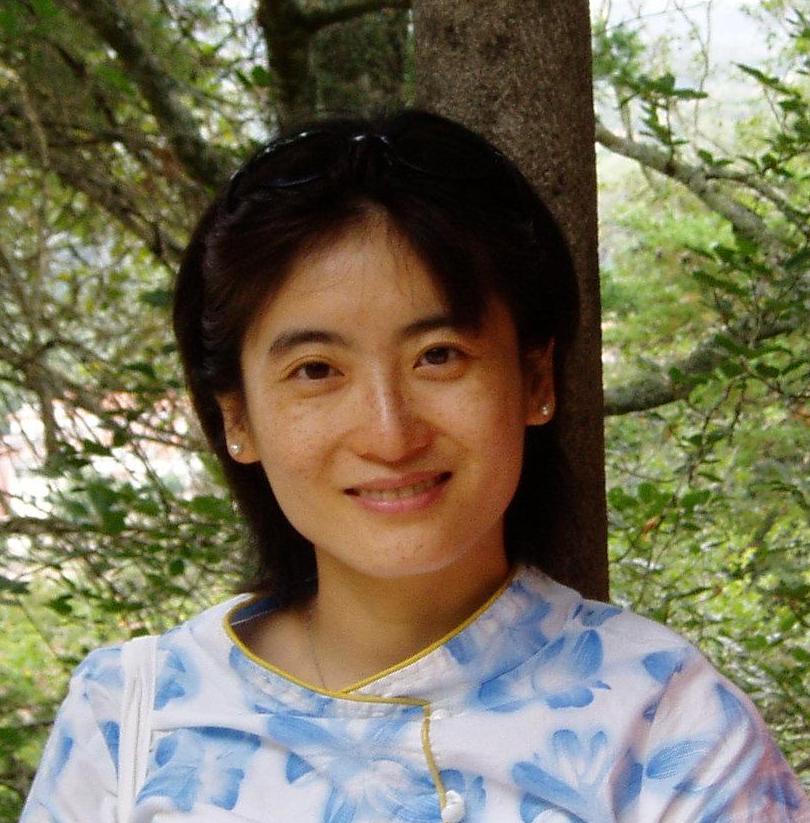