Colloquia
Our department is proud to host weekly colloquium talks featuring research by leading mathematicians from around the world. Most colloquia are held on Fridays at 4pm in ACLC, Room 010 (unless otherwise advertised) with refreshments preceding at 3:15pm in Parker Hall, Room 244.
Apr 26, 2024 04:00 PM
(please note special location--228 Parker Hall)
Speaker: Jordan Awan (Purdue)
Abstract: Privacy protection methods, such as differentially private mechanisms, introduce noise into resulting statistics which often produces complex and intractable sampling distributions. In this paper, we propose a simulation-based "repro sample" approach to produce statistically valid confidence intervals and hypothesis tests, which builds on the work of Xie and Wang, 2022. We show that this methodology is applicable to a wide variety of private inference problems, appropriately accounts for biases introduced by privacy mechanisms (such as by clamping), and improves over other state-of-the-art inference methods such as the parametric bootstrap in terms of the coverage and type I error of the private inference. We also develop significant improvements and extensions for the repro sample methodology for general models (not necessarily related to privacy), including (1) modifying the procedure to ensure guaranteed coverage and type I errors, even accounting for Monte Carlo error, and (2) proposing efficient numerical algorithms to implement the confidence intervals and \(p\)-values.
Apr 19, 2024 04:00 PM
Please note special location for colloquium--Parker 228
Speaker: Haomin Zhou (Georgia Tech)
Title: Analysis and Computation of Parameterized Wasserstein Geometric Flow
Abstract: We introduce a new parameterization strategy that can be used to design algorithms simulating geometric flows on Wasserstein manifold, the probability density space equipped with optimal transport metric. The framework leverages the theory of optimal transport and the techniques like the push-forward operators and neural networks, leading to a system of ODEs for the parameters of neural networks. The resulting methods are mesh-less, basis-less, sample-based schemes that scale well to higher dimensional problems. The strategy works for Wasserstein gradient flows such as Fokker-Planck equation, and Wasserstein Hamiltonian flow like Schrodinger equation. Theoretical error bounds measured in Wasserstein metric is established.
This presentation is based on joint work with Yijie Jin (Math, GT), Shu Liu (UCLA), Has Wu (Wells Fargo), Xiaojing Ye (Georgia State), and Hongyuan Zha (CUHK-SZ).
DMS Colloquium: Dr. Grady Wright
Apr 12, 2024 04:00 PM
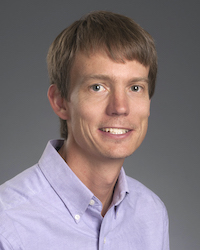
DMS Colloquium: Dr. Lateefah Id-Deen
Apr 05, 2024 04:00 PM
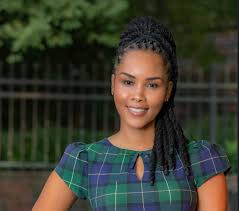
DMS Colloquium: Dr. Melinda Lanius
Mar 29, 2024 04:00 PM
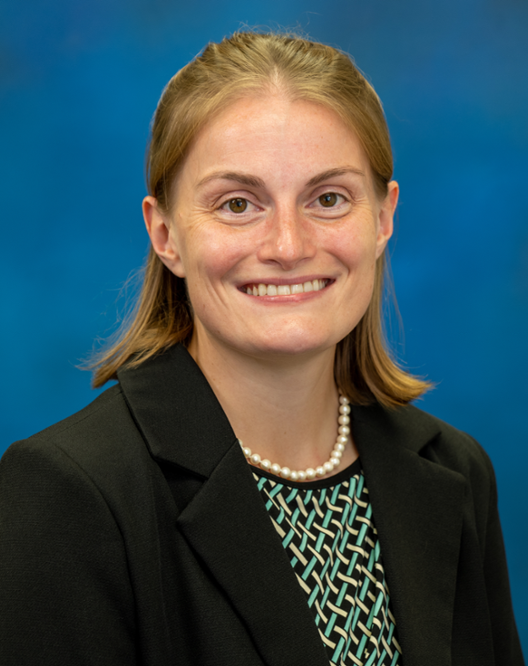
Title: Playing the villain: Approaches to validity in undergraduate mathematics education research
Abstract: In the setting of education research, a measure assigns a numerical value to represent the degree to which a target attribute appears; For example, a psychometric scale for math anxiety assigns a numerical value to indicate if an undergraduate student has high, medium, or low/no math anxiety. How do we know if a measure means what we think it means? We can collect evidence of validity! In this highly interactive talk, I will share my journey in understanding validity and the types of evidence we can use to assess the soundness of our measures. This reflective work has required me to look at my study designs unenthusiastically and critically, "playing the villain" in my own research program. Please come join me for an afternoon of fun, where we put on our critic hats and explore validity in undergraduate mathematics education research!
DMS Colloquium: Dr. Joseph Briggs
Mar 01, 2024 04:00 PM
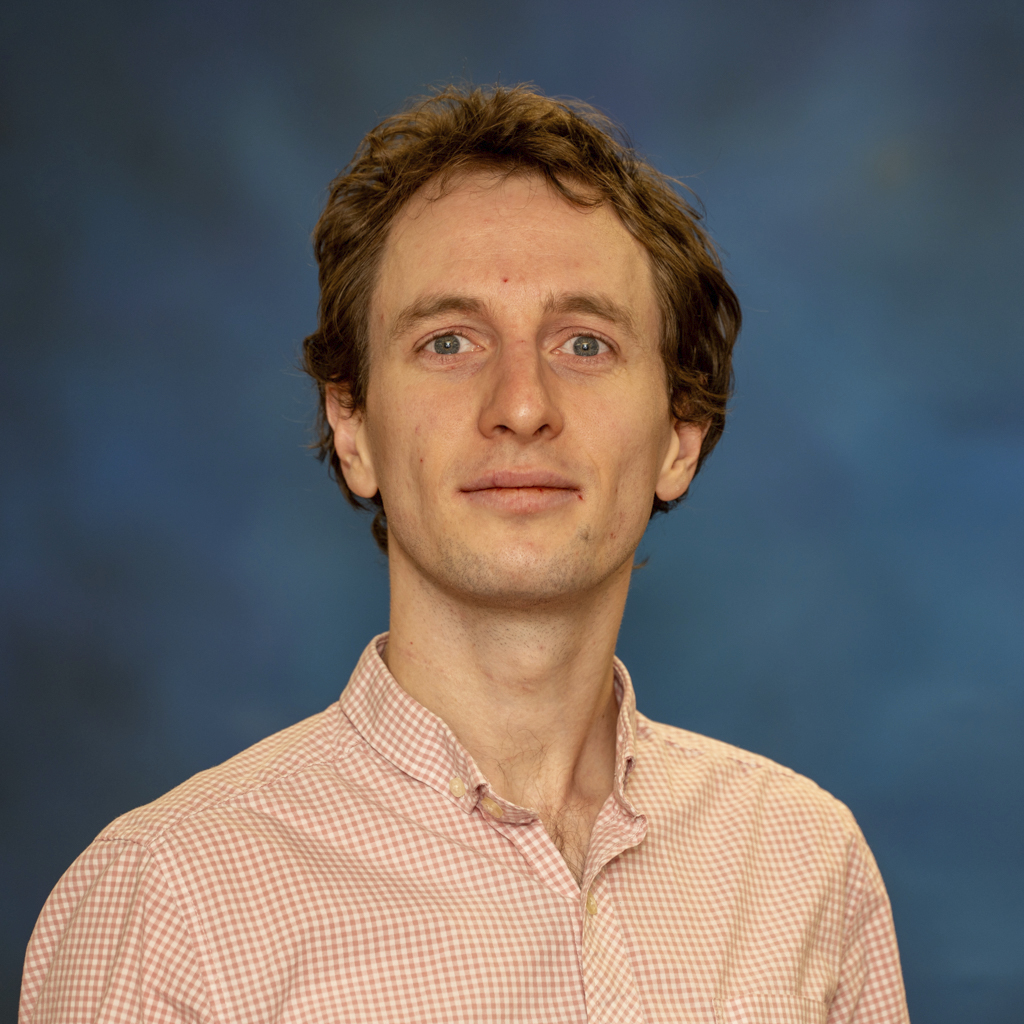
DMS Colloquium: Peter McGrath
Dec 01, 2023 04:00 PM
Refreshments 3:30 p.m., Parker 244
Speaker: Peter McGrath (North Carolina State University)
Title: Calculus of Variations and the Bending Energy of Surfaces